As the environmental pressures are mounting to move towards a net-zero carbon economy, operational efficiencies are becoming more critical on both sides of supply and demand of energy production and consumption equation. In search of more renewable and green energy sources, producers are reaching further into offshore deep ocean, large scale floating wind turbine farms, or deeper into earth for geothermal energy production. As a result, operational data should be captured using sensors; generally distributed among large geographical areas, which are difficult or costly to access. Hence, proper design and sensory communication architectures, while utilizing knowledge discovery and predictive techniques are paramount for reliable and safe operations.
With a case study of an open data set the presenters will discuss application of advanced analytics techniques for detecting multivariate outliers, anomalies, and operational non-productive time, while predicting and preventing future undesirable events (e.g., failures) based on historical data. Applications of machine learning techniques along with statistical models for distinguishing unexpected short term operational anomalies, data mining and knowledge discovery will be discussed. Additionally, as success of advanced analytics techniques depends on availability of reliable and high-quality sensory data; convergence of IT, Operation Technologies and the cloud hosting models will be positioned to provide a solid foundation for similar big data initiatives in key industries with high impact on environmental concerns.
Speakers
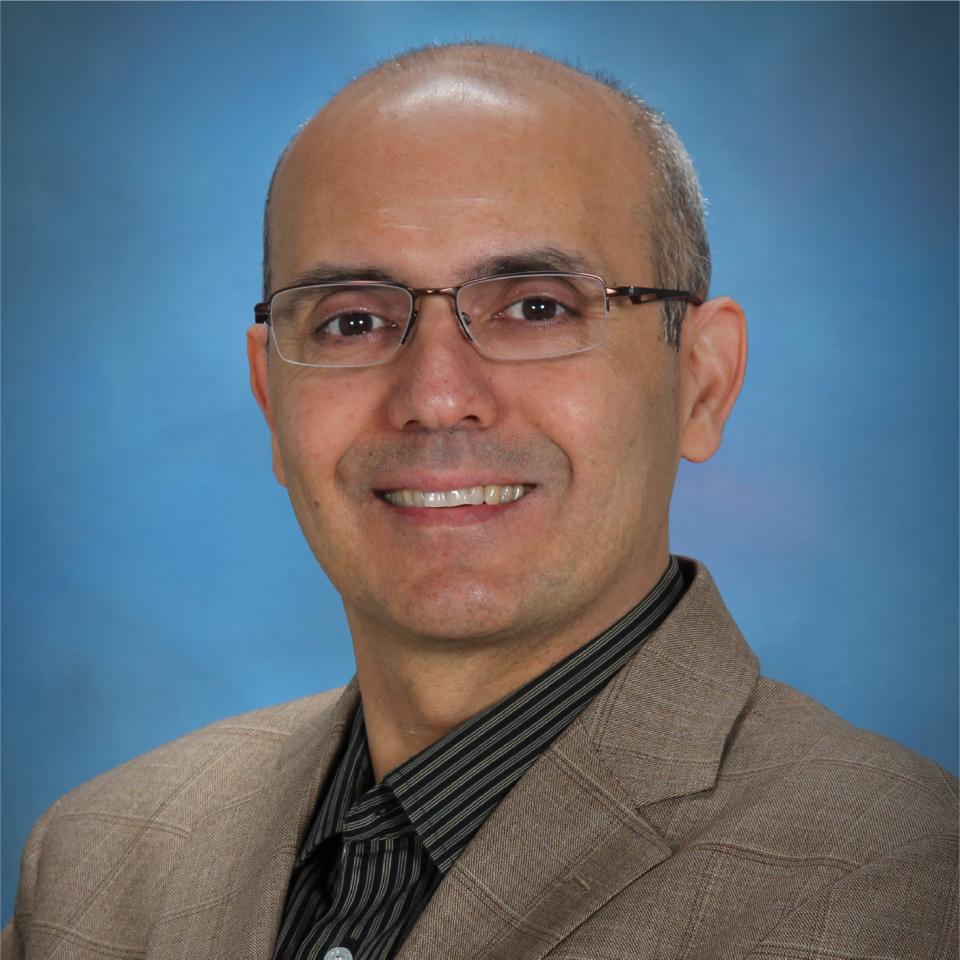
Alan Rezazdeh
Analytics Business Development Manager | Southern Alberta Institute of Technology
Alan Rezazadeh is an analytics research scientist with Applied Research and Innovation Services of SAIT Polytechnique, Calgary, Alberta. For the last 25 years Alan has been working on data governance and analytics initiatives related to energy production, distribution and sustainability, within private and public sectors. Alan earned a PhD degree in Computer Science from University of Regina, with focus on applied mathematics. His recent engagements include applications of statistical analysis and machine learning in pollution prediction, wind turbine condition monitoring as well as remote sensing techniques for greenhouse gases (methane leaks) and native hydrogen emission detection and quantification.
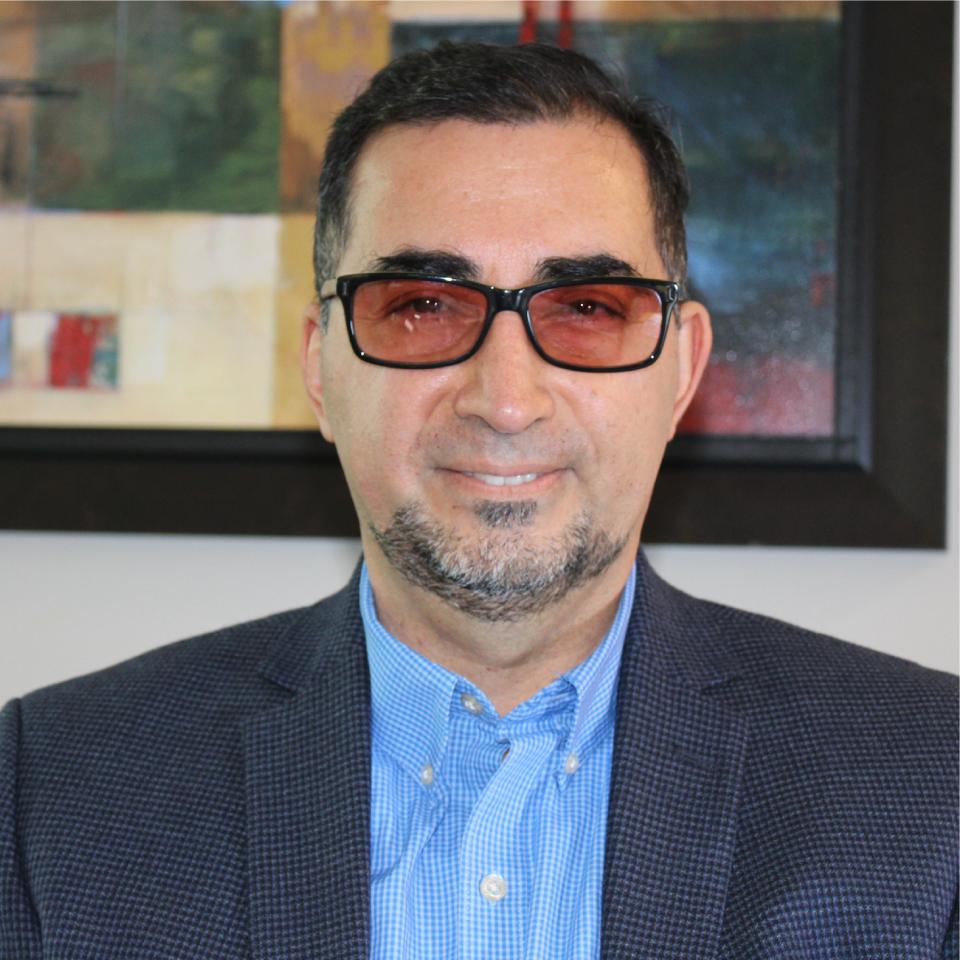
Samuel Rostam
Adjunct Professor | NYIT
Samuel Rostam is a senior technical advisor and an adjunct professor at NYIT Vancouver campus. His clients include a range of multi-faceted organizations with a complex mix of technologies from start-ups to large enterprises and governments in the US & Canada. In previous positions, Sam’s primary role has been as an Enterprise Systems Architect with experience across many platforms including distributed systems integrations and cloud computing.
Sam worked as a senior architect with the enterprise services group at Sun Microsystems, focusing primarily on EAI and modernizations and integration architecture across several industries including healthcare, insurance, banking, e-commerce, and government agencies. Additionally, for over 6 years as a trusted advisor to the WA State Correction, he was a technical lead during the modernization of an offender management system (OMNI) leveraging Web/APIs and Java Enterprise platform. Samuel is a Certified Architect in several areas that include Cloud, SOA, and Blockchain. As a certified Technical Trainer, he has conducted over 100 global technical training certification workshops, such as business architecture bootcamp, BizBok, TOGAF, Java Enterprise, Machine Learning, AI, Bigdata science, SOA Governance/Analysis, and vendor-neutral Cloud Architecture with integration design patterns.